2D-3D Interlaced Transformer for Point Cloud Segmentation with Scene-Level Supervision ICCV 2023
- Cheng-Kun Yang NTU, MediaTek
- Min-Hung Chen NVIDIA
- Yung-Yu Chaung NTU
- Yen-Yu Lin NYCU
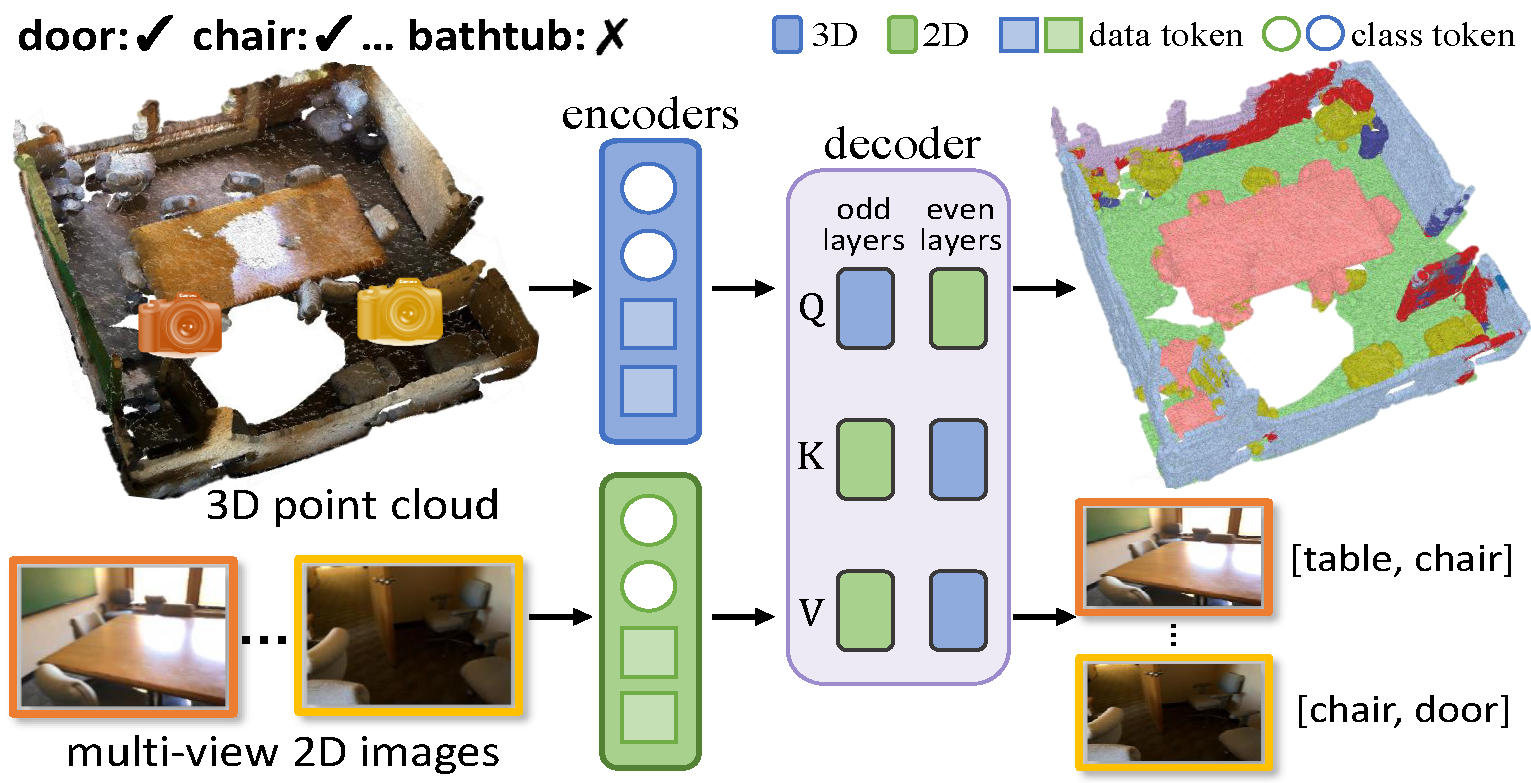
Abstract
We present a Multimodal Interlaced Transformer (MIT) that jointly considers 2D and 3D data for weakly supervised point cloud segmentation. Research studies have shown that 2D and 3D features are complementary for point cloud segmentation. However, existing methods require extra 2D annotations to achieve 2D-3D information fusion. Considering the high annotation cost of point clouds, effective 2D and 3D feature fusion based on weakly supervised learning is in great demand. To this end, we propose a transformer model with two encoders and one decoder for weakly supervised point cloud segmentation using only scene-level class tags. Specifically, the two encoders compute the self-attended features for 3D point clouds and 2D multi-view images, respectively. The decoder implements interlaced 2D-3D cross-attention and carries out implicit 2D and 3D feature fusion. We alternately switch the roles of queries and key-value pairs in the decoder layers. It turns out that the 2D and 3D features are iteratively enriched by each other. Experiments show that it performs favorably against existing weakly supervised point cloud segmentation methods by a large margin on the S3DIS and ScanNet benchmarks.
Qualitative result
MIT visual results on the ScanNet dataset
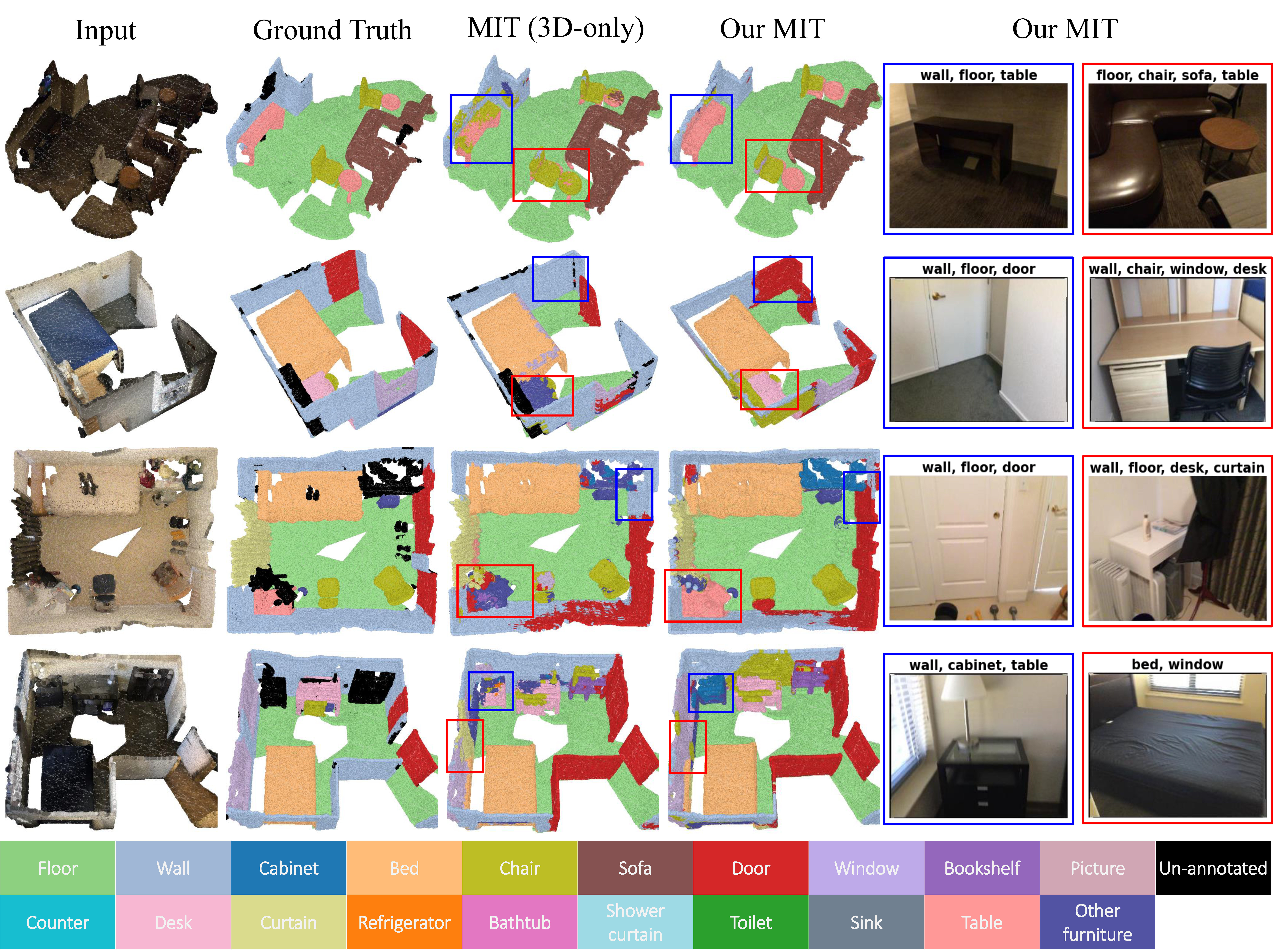
Quantitative result
Method | Supervision | Extra inputs | ScanNet | S3DIS | |||
---|---|---|---|---|---|---|---|
RGB | Pose | Depth | Val. | Test | Test | ||
MinkNet |
Fully |
- |
- |
- |
72.2 |
73.6 |
65.8 |
DeepViewAgg |
v |
v |
- |
71.0 |
- |
67.2 |
|
SemAffiNet |
v |
v |
v |
- |
74.9 |
71.6 |
|
Kewon et al. |
Scene + Image |
v |
v |
49.6 |
47.4 |
||
MIL-Trans |
Scene |
- |
- |
- |
26.2 |
- |
12.9 |
WYPR |
- |
- |
- |
29.3 |
24.0 |
22.3 |
|
MIT (3D-only) |
- |
- |
- |
31.6 |
26.4 |
23.1 |
|
MIT (Ours) |
v |
- |
- |
35.8 |
31.7 |
27.4 |
Citation
Acknowledgements
This work was supported in part by the National Science and Technology Council (NSTC) under
grants 111-2628-E-A49-025-MY3, 112-2221-EA49-090-MY3, 111-2634-F-002-023,
111-2634-F-006-012, 110-2221-E-002-124-MY3, and 111-2634-F-002-022.This work was funded
in part by MediaTek, Qualcomm, NVIDIA, and NTU-112L900902.
The website template was borrowed from Jon
Barron.